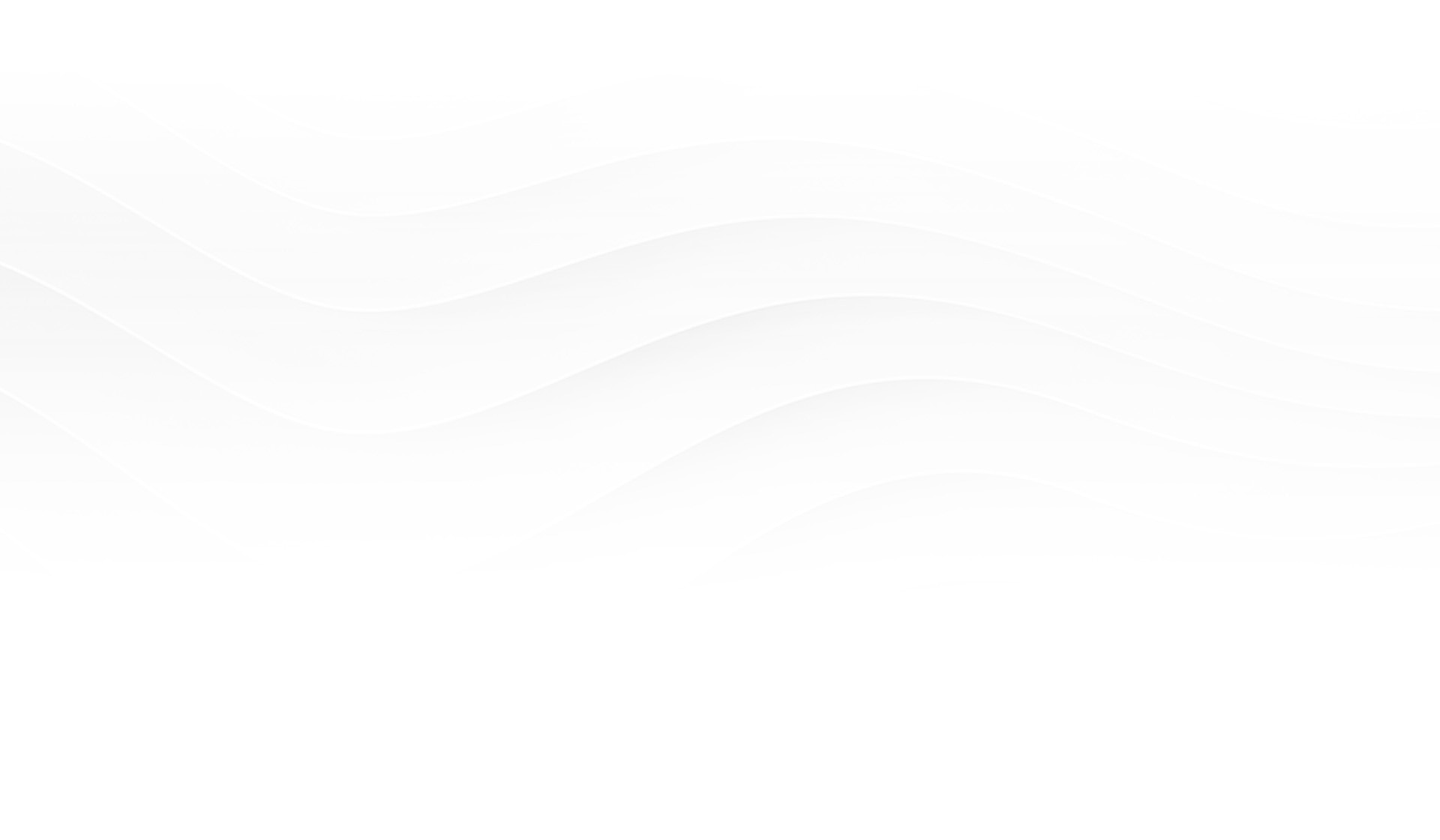
Generative AI (GenAI) What is Generative AI (GenAI)?
Generative AI, often known as Gen AI, is a specialized field within artificial intelligence that focuses on producing a wide range of material in many areas, such as text, photographs, music, audio, movies, and 3D representations. These models acquire complex patterns and structures from their training data, allowing them to generate new material with comparable properties independently. DALL-E is a generative model that can produce visuals by utilizing textual descriptions. Gen AI can perform the opposite action by generating textual descriptions for provided photos. Generative AI uses advanced algorithms and neural networks to enhance creativity, expanding the capabilities of robots in content production. AI applications encompass several fields, such as creative arts, design, entertainment, and others.
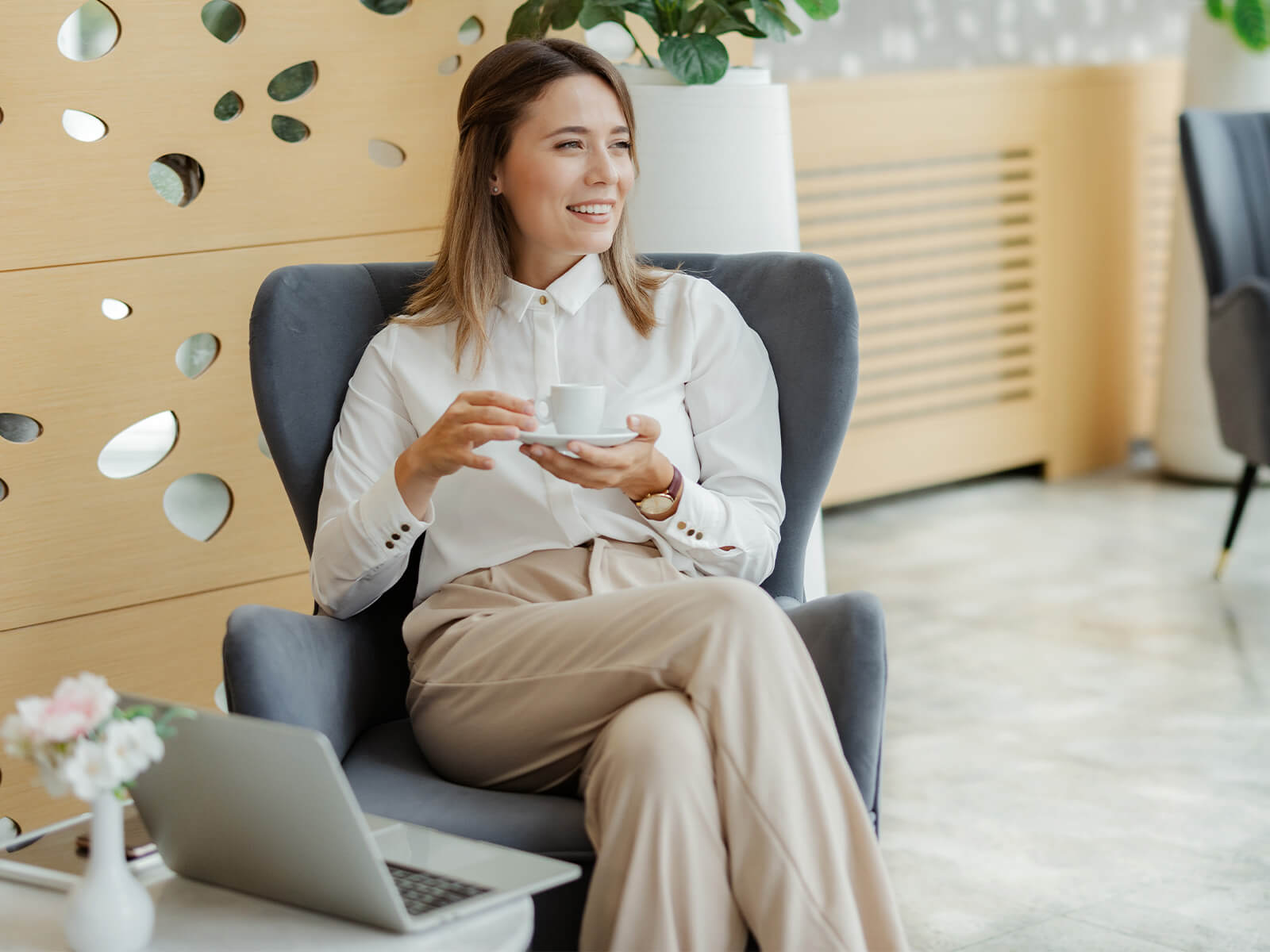
- Practical ways you can use Generative AI
- Generative AI tools
- Generative AI components
- Partner with HPE
Practical ways you can use Generative AI
Artificial Intelligence (AI) has a plethora of practical applications that can be utilized in diverse domains. Short form that can be utilized in diverse domains. that can be utilized in diverse domains. Here are some practical ways you can use generative AI:
- Chatbots for conversations: Implement chatbots that use generative AI to engage in natural language conversations, providing customer support, answering queries, and assisting users.
- Image prototyping: Use generative AI models to rapidly prototype and generate images for design concepts, art, or product ideas.
- Marketing prompts for ads: Employ generative AI to generate creative and compelling marketing prompts for advertisements, social media campaigns, or content creation.
- Short form video: Create short form videos with the help of generative AI, whether it's generating video content, automating video editing, or assisting in special effects.
- Translation of text: Utilize generative AI models to enhance language translation services, providing accurate and contextually relevant translations for various languages.
- Video into transcript: Convert video content into transcriptions using generative AI, facilitating content accessibility, and making it easier to analyze and search through video data.
- These applications showcase the versatility of generative AI in automating and enhancing various processes, ultimately improving efficiency and creativity across different industries.