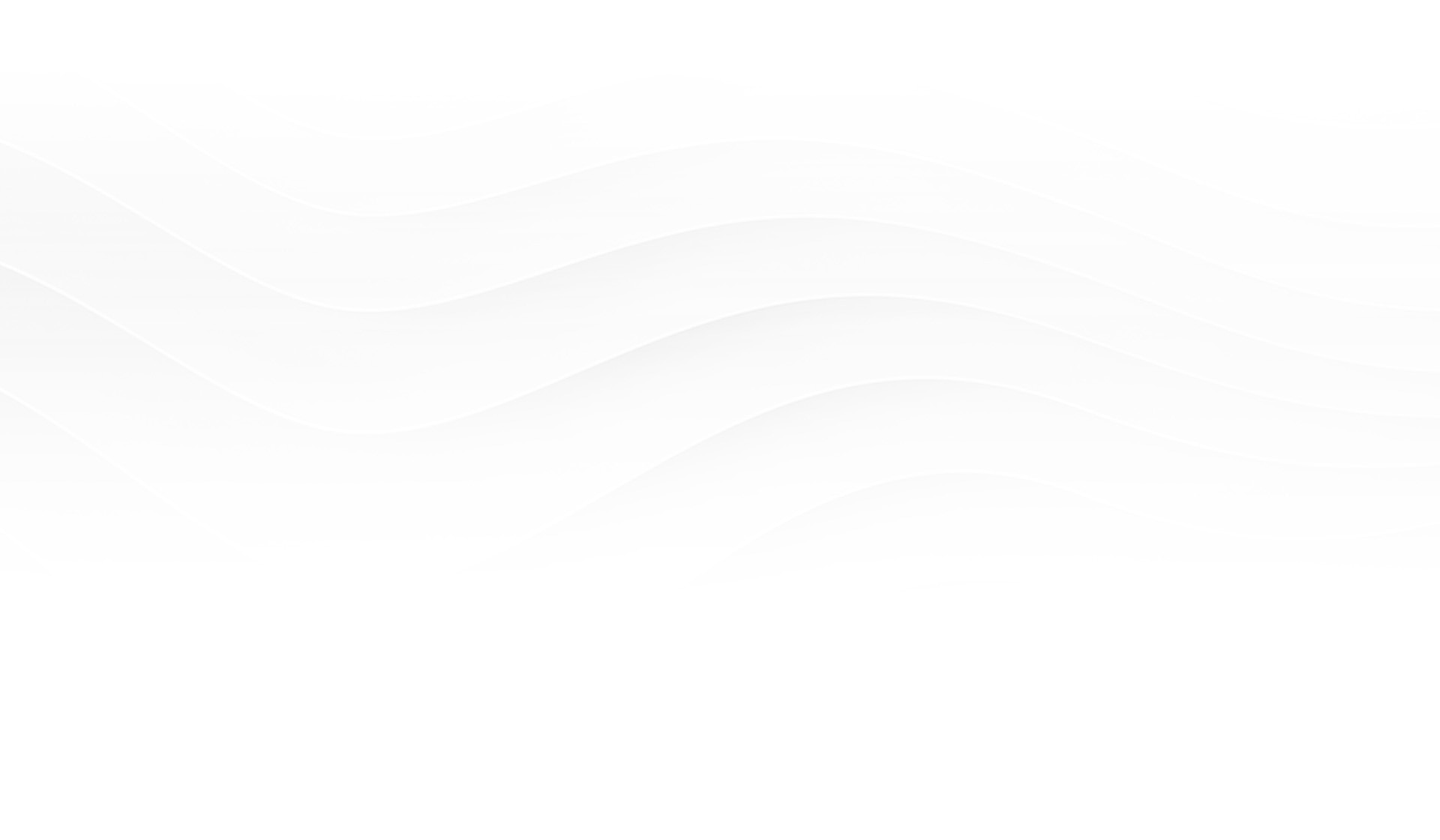
Enterprise AI What is Enterprise AI?
Enterprise AI leverages AI, machine learning, natural language processing, and predictive analytics to drive business goals and decisions. This includes industries such as finance, manufacturing, retail, tech, and the public sector.

- Enterprise AI explained
- Benefits & challenges of Enterprise AI
- Implementing enterprise AI
- HPE Enterprise AI tools
- Business Goals
How businesses use Enterprise AI
Enterprise AI is helping companies improve efficiency, decision-making, and opportunities. Here's a detailed look into how companies employ Enterprise AI across functions:
- Support and customer service:
- Chatbots and virtual assistants: 24/7 AI-powered chatbots answer questions, give help, and make suggestions.
- Customer sentiment analysis: Assessing comments, reviews, and social media interactions to enhance service.
- Sales and marketing:
- Customer Insights and personalization: AI can evaluate consumer data and behavior to tailor marketing campaigns and product suggestions.
- Predictive analytics: AI models forecast consumer behavior, sales trends, and market potential for better decision-making.
- Logistics & supply chain:
- Demand forecasting: AI optimizes inventories and reduces costs by predicting demand.
- Route optimization: AI algorithms reduce fuel expenses and speed delivery.
- Human resources:
- Recruiting: AI screens applicants, schedules interviews, and conducts initial interviews.
- Employee engagement: AI technologies track staff satisfaction and engagement to improve workplace culture and retention.
- Finance & accounting:
- Fraud detection: AI algorithms detect unnatural transaction patterns and abnormalities to avoid fraud.
- Financial forecasting: AI-driven analytics increase budgeting and forecasting accuracy.
- Development of products:
- Design and prototyping: AI helps design things, build prototypes, and simulate performance.
- Quality assurance: AI-powered quality control systems find faults and assure production quality.
- Maintenance and operations:
- Predictive maintenance: AI predicts equipment breakdowns, decreasing downtime and expenses.
- Process optimization: AI improves operational process efficiency and productivity.
- Security:
- Cybersecurity: By monitoring network traffic, AI improves threat detection and response.
- Physical security: AI-powered cameras screen for suspicious activity.
- Data management & analysis:
- Big data analytics: AI analyzes massive data sets to find trends, patterns, and insights for strategic decision-making.
- Data integration: AI technologies combine data from different sources for improved analysis and reporting.
- Healthcare:
- Diagnostics: AI analyzes medical imagery and patient data to diagnose illnesses.
- Personalized treatment: AI algorithms base treatment strategies on patient history and genetics.
- Retail:
- Inventory management: AI anticipates and automates stock reorders.
- Customer experience: AI improves in-store and online purchasing with tailored suggestions and fast checkout.
- Manage Energy:
- Smart grids: AI optimizes energy distribution and consumption, saving money.
- Renewable energy: AI anticipates, stores, and distributes renewable energy.
Enterprise AI improves productivity, lowers costs, and boosts innovation and customer engagement. AI helps companies compete in various industries.
Benefits & challenges of Enterprise AI
- Advantages of Entrepise AI
- Enterprise AI has many business benefits:
- Productivity: AI automates monotonous processes so employees can focus on higher-value tasks.
- Better judgments: AI's data-driven insights and predictive analytics enable fast and informed judgments.
- Cost savings: AI automates and predicts maintenance to optimize processes.
- Personalization: AI personalizes client interactions, increasing pleasure and loyalty.
- Scalability: AI systems help corporate development by handling massive data and transaction volumes.
- Innovation: AI helps create new goods, services, and business models.
- Risk management: AI improves security and compliance by diagnosing and managing risks.
- Challenges of Enterprise AI
However, AI implementation is challenging:
- Quality: AI needs high-quality data; insufficient or biased data could negatively impact results.
- Talent & skill gap: The talent and skill gap in AI presents a challenge for businesses seeking to implement and leverage AI technologies effectively.
- Implementation complexity: AI deployment is demanding and requires significant outcomes.
- Ethical and legal issues: AI presents prejudice and privacy concerns that must be considered.
- Change management: Employee concerns must be addressed while introducing AI.
- Initial investment: AI technologies can require substantial investment, especially for SMEs.
- Security risks: AI systems need strong security to prevent cyberattacks.
- Maintenance: AI models need constant monitoring and upgrading to be accurate.
Businesses can benefit from AI while reducing risks by solving these issues.
Implementing enterprise AI
- Set Clear Objectives: Identify business issues or opportunities where AI can add value, such as operational efficiency, customer experience, or decision-making. Perform a detailed study to rank objectives by effect and feasibility. Specify success metrics and outcomes. Ensure AI activities match company strategic goals.
- Build a Skilled Team: Get a multidisciplinary team with data science, machine learning, software development, and business domain experience to drive AI initiatives in the company. Encourage cooperation and constant learning to keep team members up to speed on AI technology and methods. Offer professional development and training to improve skills. Encourage diversity and inclusion to boost creativity and views.
- Infrastructure and governance: Ensure AI project data quality, security, and accessibility with strong data infrastructure and governance. This encompasses GDPR and CCPA compliance, data collection, storage, and processing. Data governance frameworks specify data management roles, responsibilities, and processes throughout their lifespan. Invest in data integration tools and platforms to simplify data pipelines and provide AI applications with relevant data.
- Pilot projects and proof of concepts: Use small-scale pilot projects to show how AI technologies may affect certain business operations. Validate AI application feasibility and efficacy via low-risk use cases with explicit success criteria. Collaborate with stakeholders to acquire pilot feedback and insights. Iterate on lessons learned and improve AI models or algorithms before expanding. Communicate pilot project findings and lessons to develop organizational trust and support for AI adoption.
- Integration and scalability: Integrate AI technologies with existing systems and workflows for smooth adoption and scaling. Develop a strategy for adopting AI technology across departments or business units to meet organizational strategic goals. Ensure interoperability with current IT infrastructure to reduce interruption and enhance ROI. Use cloud services and scalable architectures to handle expanding data and user needs. Use monitoring and performance management to track AI solution efficacy and scalability.
- Continuous assessment and Optimization: Create an AI model and application assessment and optimization process. Identify optimization opportunities by monitoring performance indicators and user comments. Use A/B testing, user feedback loops, and model retraining to improve AI accuracy, robustness, and relevance. Keep up with AI research and technology to add new skills and stay competitive in the fast-changing industry. Create an innovative and experimental culture to explore new AI use cases and economic value-generating potential.
- Ethics and responsible use of AI: Prioritize ethics and responsible use of AI throughout AI development. Address bias, fairness, openness, and accountability to reduce risks and ensure AI system trustworthiness. Ethical AI principles and frameworks should govern AI solution design, development, and deployment. Ask data subjects, consumers, workers, and regulators about ethical AI concepts and practices. Promote responsible AI governance and compliance to uphold ethics and public trust in AI technology.
HPE Enterprise AI tools
HPE (Hewlett Packard Enterprise) offers a range of AI tools and solutions designed to help organizations streamline and improve AI operations. Here's how each tool helps businesses:
- Consultation and expertise: HPE AI Services offers AI strategy, implementation, and optimization assistance from AI professionals.
- Custom AI solutions: HPE uses advanced algorithms and approaches to create AI solutions for unique business needs.
- Data science services: HPE AI Services helps organizations construct predictive models and gain actionable insights from their data.
- AI implementation support: HPE helps enterprises implement, integrate, and maintain AI technologies.
- Secure and scalable infrastructure: HPE Private Cloud for AI runs AI workloads securely and reliably.
- Data management and governance: HPE provides private cloud data management and governance capabilities to ensure compliance and data integrity.
- Resource optimization: HPE's private cloud solutions improve AI workload resource allocation to increase utilization and save expenses.
- Flexibility and control: HPE Private Cloud for AI lets enterprises create settings and scale resources to meet demand.
- HPE Enterprise Gen AI Solution:
- Next-generation AI capabilities: HPE's enterprise Gen AI solution addresses organizations' changing demands with enhanced AI capabilities.
- Predictive analytics: HPE helps organizations predict market trends, consumer behavior, and operational performance using predictive analytics.
- Personalization and consumer Insights: HPE's Gen AI solution uses AI to understand consumer preferences, behavior, and sentiment to tailor customer experiences.
- Automation and efficiency: HPE's enterprise solution automates company processes and operations, increasing productivity and efficiency.
In conclusion, HPE's AI tools and solutions let organizations use AI technology for strategy, consultancy, infrastructure, and execution to innovate, compete, and achieve goals.
Business Goals
This section is based on how Market leaders and business decisions markers are looking to utilize enterprise AI
What specific business problems can AI help us solve and how can we identify the highest-value opportunities for AI implementation within our organization?
AI can help solve business problems such as:
Problem
Risk management: Effective risk management is crucial, yet traditional methods lack the agility needed to respond swiftly to changing market conditions and emerging risks.
Solution
Enhance risk management: AI enhances agility in risk management by quickly responding to changing market conditions and emerging risks. This allows financial institutions to better anticipate and mitigate potential threats, ensuring more robust and proactive risk management.
Problem
Operational efficiency: Maintaining efficiency in operations is difficult amidst growing volumes of transactions and data, leading to increased operational costs and slower service delivery.
Solution
Improve operational efficiency: AI improves operational efficiency by automating routine tasks and optimizing workflows. This reduces operational costs, speeds up service delivery, and allows human resources to focus on more complex and value-added activities.
