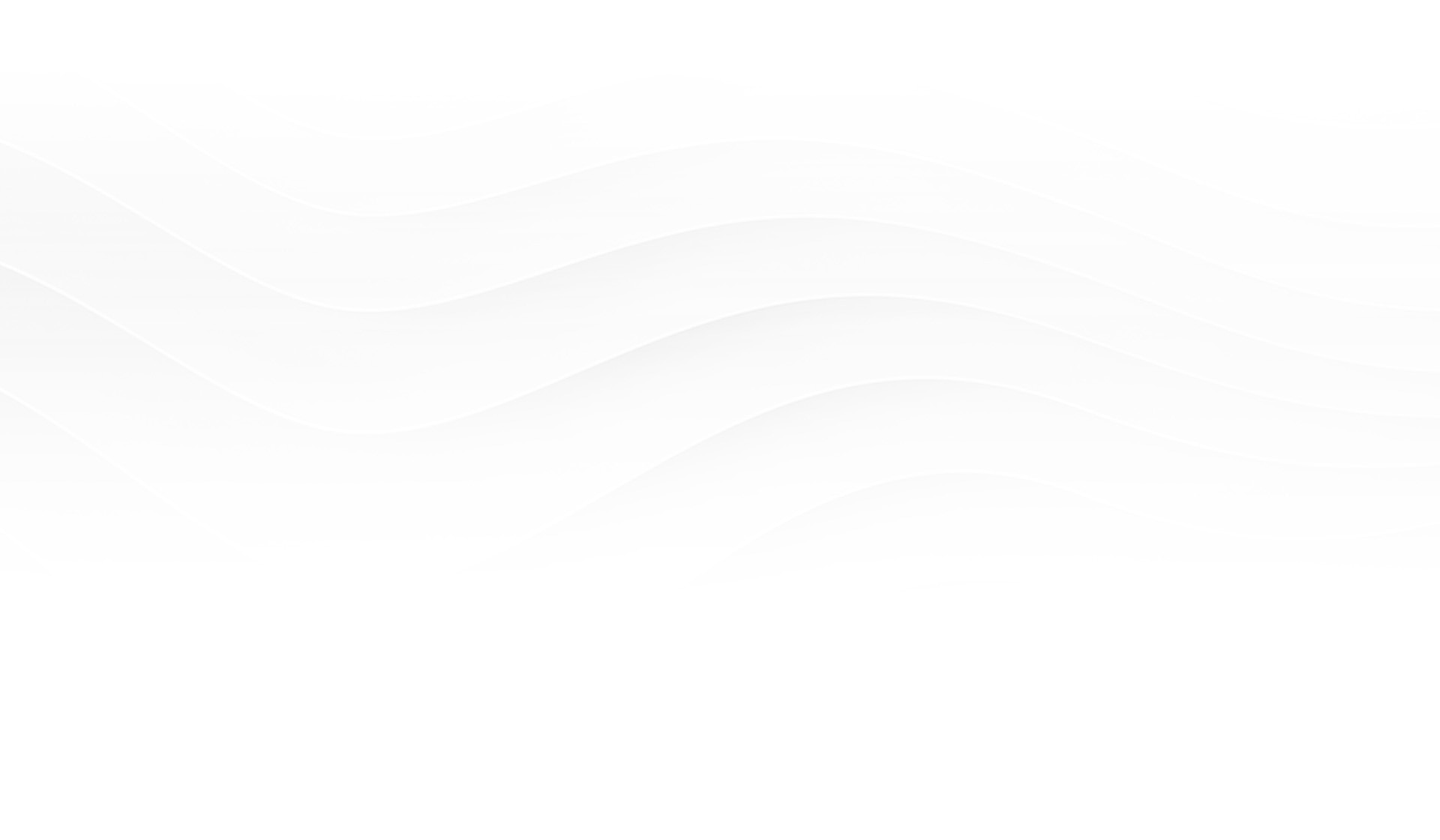
AI Data Center Networking What is AI data center networking?
Artificial intelligence (AI) optimizes and manages data center networking operations. By evaluating data and making real-time choices, AI algorithms and machine learning models simplify network administration activities and increase performance, scalability, security, and operating expenses.

- AI data center networking breakdown
- How does AI data center networking work?
- Challenges and considerations for AI data center networking
- AI data center networking key considerations
- Resources for AI data center networking and how can HPE help
AI data center networking breakdown
AI data center networking improves data center network efficiency, performance, and administration. Here's a breakdown:
Definition and explanation
Machine learning algorithms and AI-driven analytics enhance network operations in data center networking. This includes:
- Network management: AI can automate and optimize network settings, routing, and resource allocation using real-time data and predictions.
- Predictive maintenance: AI can identify difficulties and bottlenecks by studying network traffic patterns and performance indicators, minimizing downtime.
- Security: AI can identify network traffic irregularities that suggest security risks or assaults, speeding reaction times, and improving cybersecurity.
- Performance optimization: AI can dynamically alter network parameters to optimize workload and application performance through constant monitoring and analysis.
Benefits and advantages
AI in data center networking has many benefits:
- Improved efficiency: AI algorithms dynamically alter network settings to optimize traffic, minimize latency, and boost efficiency.
- Scalability: By managing resources depending on demand and workload, AI-driven automation improves data center scalability.
- Cost savings: AI can reduce network maintenance and administration expenses by automating regular jobs and optimizing resource use.
- Enhanced security: AI can detect and respond to threats in real-time, reducing network breaches and attack risks.
- Predictive capabilities: AI's predictive analytics allow data centers to build and maintain networks based on anticipated demands and concerns.
AI data center networking transforms network infrastructure management and optimization using machine learning and AI to improve efficiency, scalability, security, and cost.
How does AI data center networking work?
AI data center networking uses algorithms and technologies to improve performance, efficiency, and security. How it works:
Overview of AI algorithms and technologies used:
- Machine learning (ML): ML algorithms find trends, forecast traffic, and optimize resource allocation in historical and real-time network data. Supervised learning can categorize network traffic for security, whereas unsupervised learning can discover abnormalities.
- Deep learning: Deep learning models, like neural networks, perform complicated tasks, such as NLP in network logs, picture identification in network topology visualization, and sensor data-based network failure prediction.
- Reinforcement learning: Updates network routing and resource allocation based on network circumstances and performance goals.
- AI-driven analytics: AI-powered analytics tools analyze massive network data to find anomalies, optimize performance, and forecast maintenance.
The impact of AI on network performance, efficiency, and security optimization:
Performance optimization:
- Dynamic resource allocation: AI algorithms optimize network resources (bandwidth, computing power) based on traffic and workload.
- Traffic engineering: AI optimizes traffic routing and load balancing to reduce latency and boost crucial application throughput.
Efficiency:
- Automation: AI automates configuration management, troubleshooting, and policy enforcement, saving IT resources and decreasing error.
- Data center energy efficiency: AI algorithms manage cooling systems and workload allocation to optimize energy use.
Security:
- Threat detection: AI analyzes network traffic patterns to identify abnormalities and security risks, including DDoS assaults and malware, and speeds threat response and mitigation.
- Behavioral analysis: AI can detect anomalous activity that may suggest unwanted access or compromised equipment.
Predictive maintenance:
- AI models anticipate network failures and performance deterioration based on past data and proactive monitoring, enabling preventative maintenance to reduce downtime.
- Fault prediction: AI models use historical data and proactive monitoring to forecast possible network faults or performance deterioration, allowing for preventative maintenance and reducing downtime.
The use of modern algorithms and technologies to optimize network operations, increase efficiency, security, and predictive capacities has transformed how data centers operate and maintain their networks.
Challenges and considerations for AI data center networking
Common challenges and obstacles in using AI in data center networking:
- Data quality and availability: AI models need extensive, high-quality data. Inconsistent, incomplete, or degraded data can hinder AI systems.
- Integration with existing systems: Integrating AI technologies into legacy network infrastructure can be difficult and can require considerable adjustments or improvements to existing systems.
- Complexity and skill gaps: Implementing and managing AI-driven solutions can require IT workers with specific capabilities.
- Cost: AI technology and infrastructure can be expensive to implement, which may deter specific organizations.
- Security and privacy risks: AI requires processing sensitive data, which raises data security and privacy risks.
- Interoperability issues: Integrating AI technologies from multiple suppliers is difficult.
- Trust and transparency: Building transparency and trust in AI algorithm judgments can be challenging.
Best practices and strategies for overcoming challenges:
- Data quality: Implement strong data governance standards to assure data quality, consistency, and completeness. Data cleaning and preprocessing increase data quality.
- Phased integration: Begin with trial projects and gradually integrate AI into current systems. This helps discover difficulties and alter techniques before full adoption.
- Training and skills development: Train IT professionals in AI and data science. Consider recruiting or consulting AI professionals to fill skill shortages.
- Cost management: Consider cloud-based AI services and cost-effective AI solutions to decrease upfront expenditure. Use AI to optimize network operations for long-term savings.
- Increasing security and privacy: Provide strong protection for AI data. Comply with data privacy laws and use privacy-preserving AI models.
- Promote interoperability: Use AI solutions that follow industry standards and open protocols. Coordinate with vendors to guarantee compatibility and integration.
- Build trust and transparency: Use explainable AI to make AI decisions transparent. Communicate AI's pros and cons to stakeholders to establish trust.
The future of AI data center networking:
- AI-driven network automation: AI will improve network automation, eliminating manual involvement and improving operational efficiency.
- Edge AI: As edge computing expands, AI will analyze data locally at the network edge, lowering latency and boosting real-time decision-making.
- AI for cybersecurity: Advanced threat detection, real-time anomaly identification, and automated incident response will improve network security.
- 5G and beyond: AI-driven network management will help 5G and future networks handle complexity and data volumes.
- Self-optimizing networks: AI will enable networks to alter settings, forecast faults, and optimize performance without human involvement.
- Sustainability: AI will optimize energy and cooling systems in data centers, decreasing environmental effects.
- AI-enhanced network analytics: Advanced AI analytics will improve decision-making by revealing network performance, user behavior, and upcoming patterns.
AI implementation in data center networking is complex, but strategic approaches and best practices might assist. AI data center networking is poised for automation, security, and efficiency.
AI data center networking key considerations
Key considerations for organizations planning to adopt AI in their data center networks
- Assess business needs and objectives: Understand the specific goals and objectives for adopting AI in data center networking. Define success as improving efficiency, security, cost savings, or scalability.
- Evaluate current infrastructure and readiness: Assess hardware, software, and data architecture for AI integration readiness. Identify any gaps or areas that can require improvements or modifications.
- Data quality and availability: Provide high-quality data for AI model training and decision-making. Data governance policies provide data integrity, security, and compliance.
- Security and privacy considerations: When deploying AI solutions, prioritize cybersecurity and data privacy. Develop secure AI systems that meet norms and requirements.
- AI integration and compatibility: Create a thorough integration plan to integrate AI into network systems smoothly. Think about legacy infrastructure compatibility and future technology interoperability.
- Skills and training: Assess the company's AI skills and identify gaps. Help it professionals learn how to manage and use AI-driven technologies.
- Start with pilot projects: Test AI applications using modest pilot projects in real-world circumstances. Pilot programs test AI systems, uncover issues, and improve implementation tactics before deployment.
- ROI and cost: Evaluate ROI and TCO for AI deployment. Consider infrastructure, software licenses, maintenance, and training costs.
- Vendor selection and partnerships: Select reputable suppliers and technology partners with demonstrated AI and data center networking competence. Collaborate closely to align with company goals and harness vendor support for effective implementation.
- Monitoring and continuous improvement: Track AI solutions' commercial results using metrics and KPIs. Continuously improve through data-driven assessments, updates, and optimizations.
By addressing these characteristics, enterprises can plan and implement AI in their data center networks to maximize performance, efficiency, and security while minimizing risks.
Resources for AI data center networking and how can HPE help
HPE (Hewlett Packard Enterprise) provides various tools, technologies, and resources that can considerably help with AI application in data centers. HPE can aid in several ways:
- AI-driven networking solutions: HPE's AI-optimized networking solutions streamline data center data transit and processing. AI applications demand high bandwidth and low latency, thus their networking gear and software are optimized.
- Compute and Storage Infrastructure: HPE provides AI-optimized computing and storage. Servers with GPUs or TPUs are used for faster machine learning algorithm processing.
- AI management and operations: HPE offers AI-specific solutions for installing, monitoring, and managing AI models and infrastructure. This comprises model training, inference, and lifecycle management tools.
- Consulting and support services: HPE helps companies create and execute customized AI solutions. Their support services assure AI infrastructure stability and performance in production scenarios.
- AI research and innovation: HPE invests in AI research and innovation to build data center AI technologies and solutions.