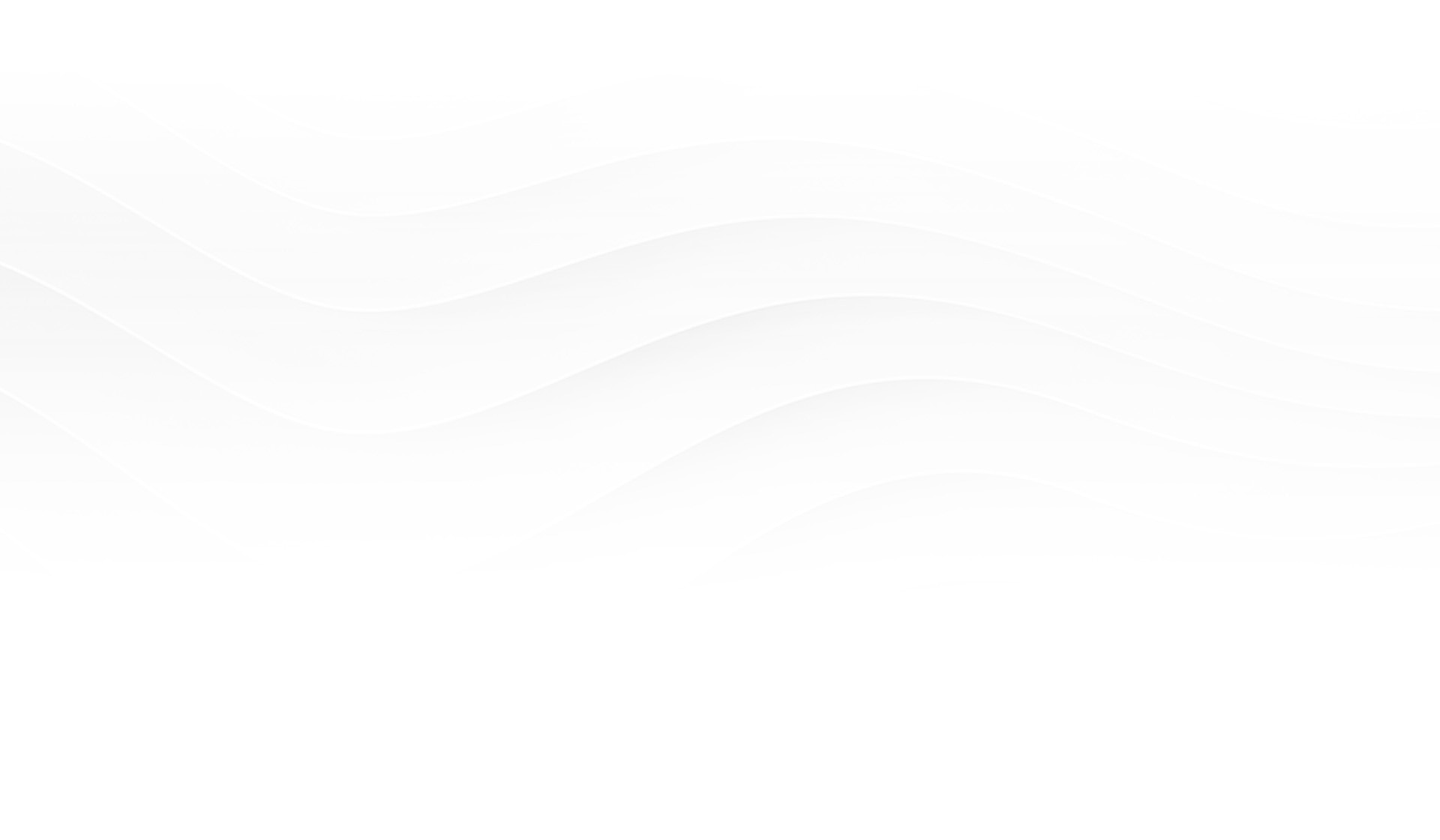
Unstructured data What is unstructured data?
Unstructured data, in the context of data storage, refers to information that varies widely in terms of format and content. It includes file and object data and has a major role in artificial intelligence (AI). This type of data does not fit neatly into predefined data models which makes storing, retrieving, and analyzing it challenging. Unstructured data is often qualitative and comes in various formats, such as emails, social media posts, articles, photos, graphics, recordings, podcasts, movies, logs, and Internet-of-Things (IoT) streams and is often stored in its native format. It accounts for a significant portion of the world's data and requires advanced tools like natural language processing (NLP), image recognition, and AI-driven analytics to derive meaningful insights.
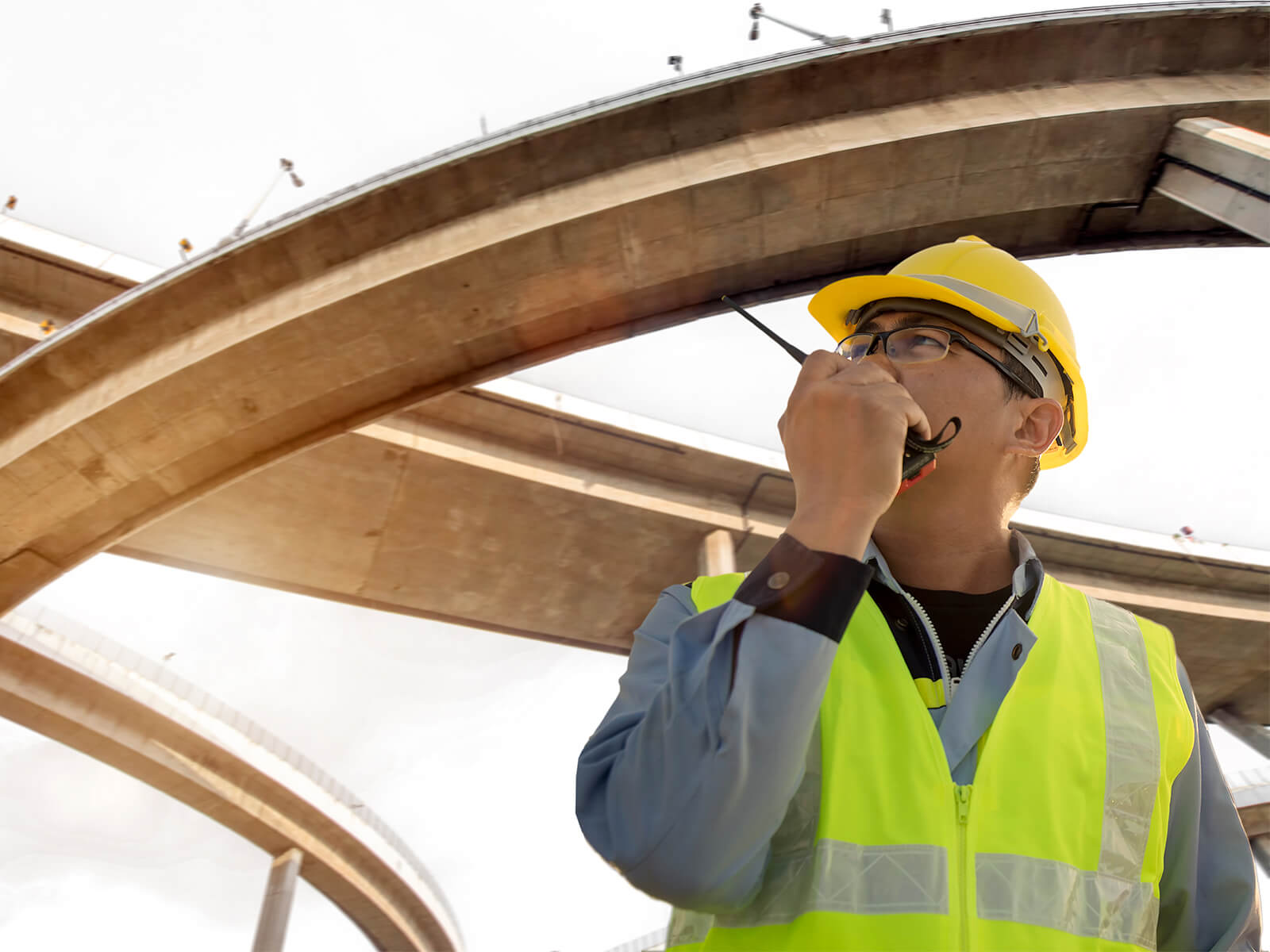
- What are examples of unstructured data?
- What are AI opportunities for unstructured data?
- How can HPE help with unstructured data?
What are examples of unstructured data?
Information without a format or organizational framework is difficult to store and handle in typical databases. This diversified data comes from many sources and forms:
Emails, social media posts, blog articles, customer reviews, chat logs, PDFs, and Word and Excel files: This data can reveal useful insights but requires NLP technologies to examine.
Multimedia: Photos, YouTube videos, podcasts, and voice recordings are included. Image recognition, video analysis, and speech-to-text transcription increasingly use these formats.
Sensors and IoT devices: Fitness trackers, smart home temperature and activity sensors, and industrial equipment machine records are examples. This data typically needs real-time processing and complex analytics.
Internet: HTML pages, clickstream navigation patterns, and web scraping data are included. Use these sources to monitor user behavior, optimize websites, or gain market insight.
Contact center transcripts, open-ended survey replies, and legal filings: These data are crucial for customer service, market research, and legal analytics, but they need complex algorithms to analyze.
What are AI opportunities for unstructured data?
AI offers huge prospects for making sense of unstructured data, which makes about 80% of global data. AI can reveal insights from text, photos, audio, and video data that doesn't fit into standard databases.
Natural Language Processing (NLP) can analyze documents, social media, and consumer feedback to discern sentiments, summarize material, and identify essential elements. These features enable chatbots, virtual assistants, and content classification, improving company communication and workflows.
AI allows facial recognition, object identification, and video synopsis for surveillance, medical imaging, and content control. The same techniques translate spoken words to text, allowing automatic transcription and voice recognition, and analyze vocal tone for emotional insights.
AI is also very good at organizing links between concepts and extracting metadata from unstructured material to create knowledge graphs. These strategies increase searchability and enable semantic search engines for more accurate and contextual results. Unstructured data is used to tailor recommendations based on user preferences, reviews, and multimedia uploads.
AI helps diagnose and treat patients by extracting information from medical pictures and clinical papers. Customer support analytics software examines chat records to find feedback trends and enhance service. Predictive analytics leverages AI techniques to reveal trends and anomalies that assist fraud detection and market analysis decisions. AI detects text and visual biases and monitors communication data for regulatory infractions, improving compliance and ethics. These applications promote standards and fairness, thus helping AI gain meaningful insights that enable businesses to derive actionable plans and drive innovation.
How can HPE help with unstructured data?
HPE offers a variety of products and services for unstructured data, including:
- HPE Alletra Storage MP X10000: A fast object data storage solution that unleashes the power of your unstructured data with scalable, high-performance, and simple management to drive innovation and accelerate time to value.
- HPE Greenlake for File Storage: A file data storage solution that accelerates AI and other data-intensive workloads with enterprise performance, simplicity, and enhanced efficiency, all at AI scale. It offers an end-to-end HPE GreenLake experience for file data storage and management.
- HPE Ezmeral: An integrated platform for processing and analyzing unstructured data. It supports data lake architectures, advanced analytics, and machine learning workflows, making it easier to extract actionable insights from diverse sources like text, images, and video data.
- HPE GreenLake: With its as-a-service model, HPE GreenLake offers scalable, cloud-like solutions for managing unstructured data. It includes storage, analytics, and AI-driven processing services, providing businesses with a flexible and cost-efficient way to handle their data.
- HPE AIOps with Data Services Cloud Console: A unified management control plane that includes AI-driven predictive analytics to manage and optimize structured data. It helps businesses ensure the reliability, performance, and efficiency of their data storage systems by proactively identifying and resolving potential issues.
- HPE StoreOnce: HPE StoreOnce provides comprehensive data protection for unstructured data through efficient backup, recovery, and deduplication capabilities. Its built-in encryption and access controls ensure the security and integrity of sensitive information.
- HPE Partnerships with AI Ecosystems: HPE collaborates with leading AI frameworks like Apache Hadoop, TensorFlow, and Spark to enhance its platforms. These partnerships enable enterprises to build advanced AI models for applications such as image recognition, natural language processing, and customer insights.
HPE’s product lineup and partnerships offer end-to-end solutions for storing, managing, analyzing, and securing unstructured data, empowering businesses to maximize their data’s value.
Unstructured data vs. structured data
Features | Unstructured data | Structured data |
---|---|---|
Format | Lacks a predefined format or organizational structure | Organized into a predefined schema (e.g., rows and columns in a database) |
Storage | Stored in data lakes, NoSQL databases, or file or object storage systems | Stored in relational databases (e.g., SQL) |
Examples | - Social media posts - Images, videos, audio files - Email content | - Customer data (name, age, email) in a CRM - Inventory data in Excel |
Querying | Requires a file or object storage system and specialized tools such as AI, NLP, or machine learning for analysis | Easy to query using SQL or similar tools |
Volume | Usually larger in size and growing rapidly in number of files and/or objects | Typically smaller and more manageable |
Analysis | Requires advanced analytics techniques, including AI and machine learning | Straightforward to analyze with conventional BI tools |
Applications | Sentiment analysis, image recognition, video analytics, trend forecasting | Financial reporting, inventory management, operational databases |
Flexibility | Highly flexible: Can handle diverse and evolving data formats | Inflexible: Schema changes require significant adjustments |
Data sources | Social media platforms, IoT devices, emails, multimedia content | Transactional systems, structured surveys |