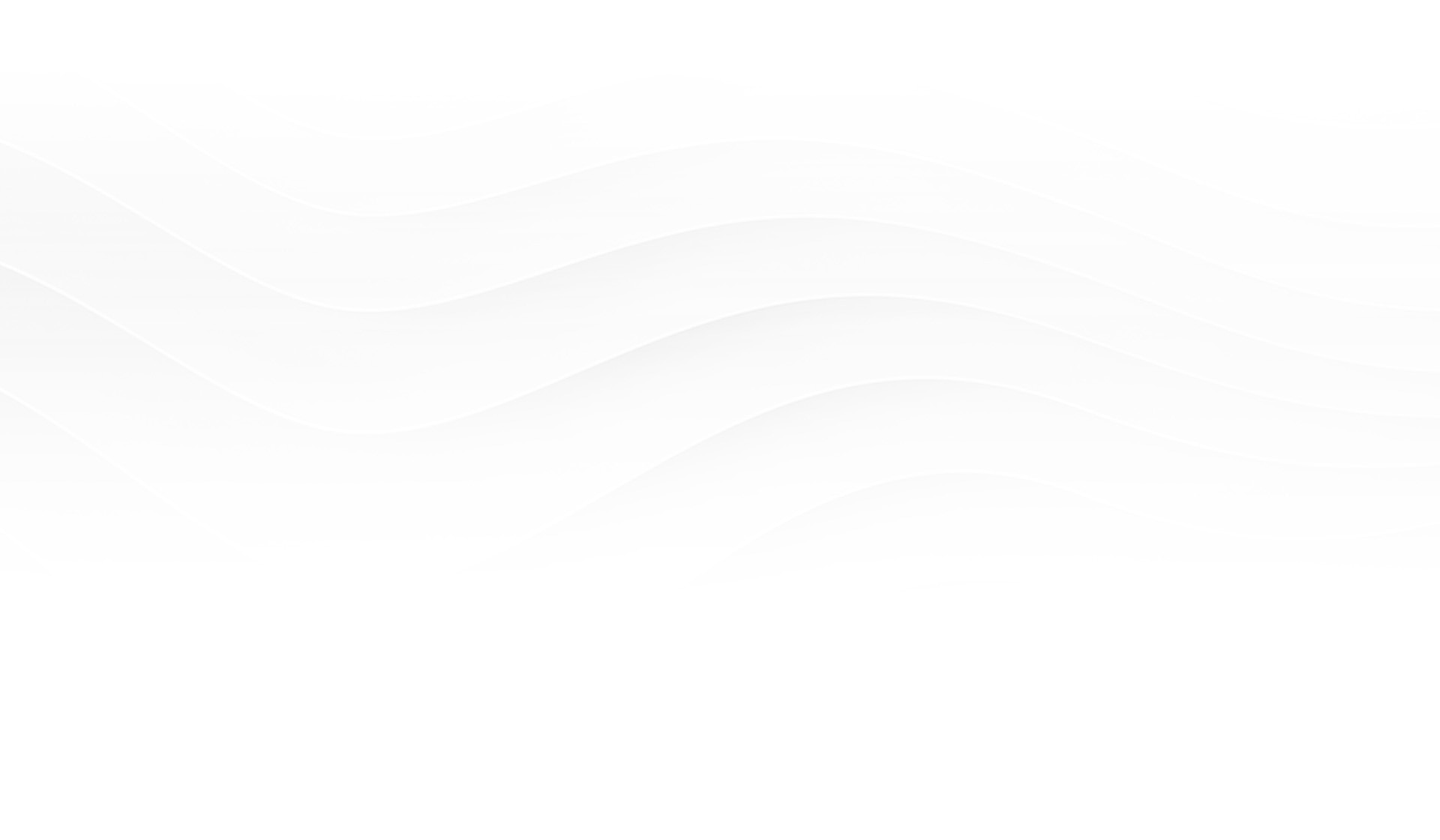
Fine-Tuning What is Fine-Tuning (AI)?
Fine-tuning in machine learning is the act of changing a pre-trained model's parameters to meet a specific task or dataset. The model is retrained on target task-related data while retaining its previous expertise.

- How does fine tuning work?
- The process of fine-tuning
- Fine-tuning with HPE
How does fine-tuning work?
Fine-tuning is like transfer learning, where the model uses its expertise to perform better on a related job. Fine-tuning a pre-trained model yields better outcomes with less computing resources and training time than starting from scratch. It is vital to contemporary machine learning workflows since it is utilized in natural language processing and computer vision to adjust models to new tasks or datasets.
Fine-tuning vs. RAG
Aspect | Fine-tuning | RAG (Retrieval-Augmented Generation) |
---|---|---|
1. Methodology | Adjusts pre-trained model parameters for specific tasks or datasets. | Utilizes a retrieval mechanism to augment generation tasks, combining retrieval and generation models. |
2. Training Data | Requires task-specific training data for fine-tuning. | Can leverage large-scale text corpora for both retrieval and generation components. |
3. Adaptability | More adaptable to a wide range of tasks and domains. | Primarily suited for tasks involving generation with contextual information retrieval. |
4. Performance | Can achieve high performance with task-specific fine-tuning. | Performance is highly dependent on the quality and relevance of retrieved information. |
5. Use Cases | Widely used in various domains such as NLP, computer vision, etc. | Particularly beneficial for tasks like question answering, dialogue systems, and content generation requiring contextual information. |