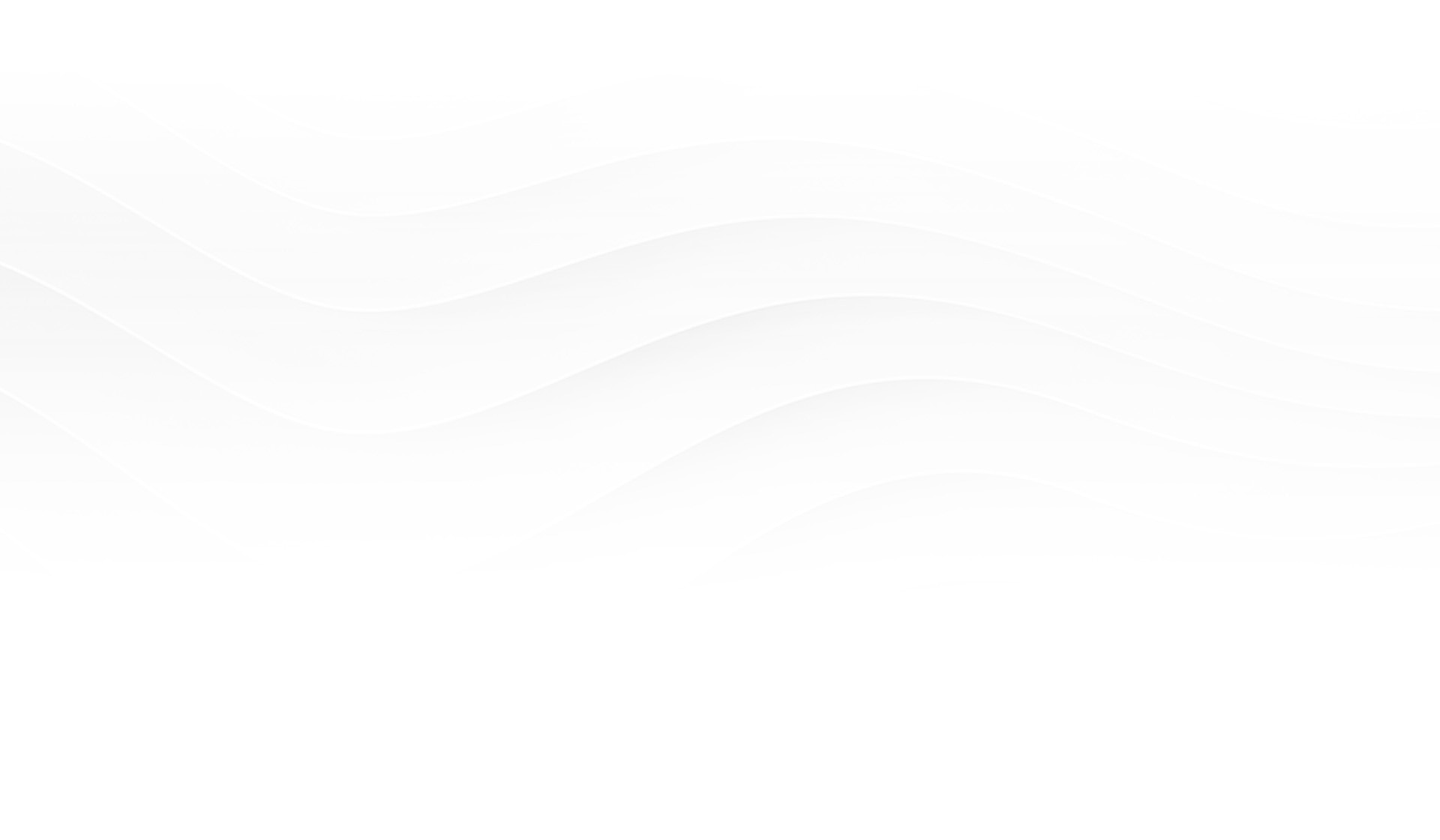
Retrieval-Augmented Generation What is Retrieval-Augmented Generation?
Retrieval-Augmented Generation (RAG) improves natural language interpretation and production by combining retrieval-based and generative models.
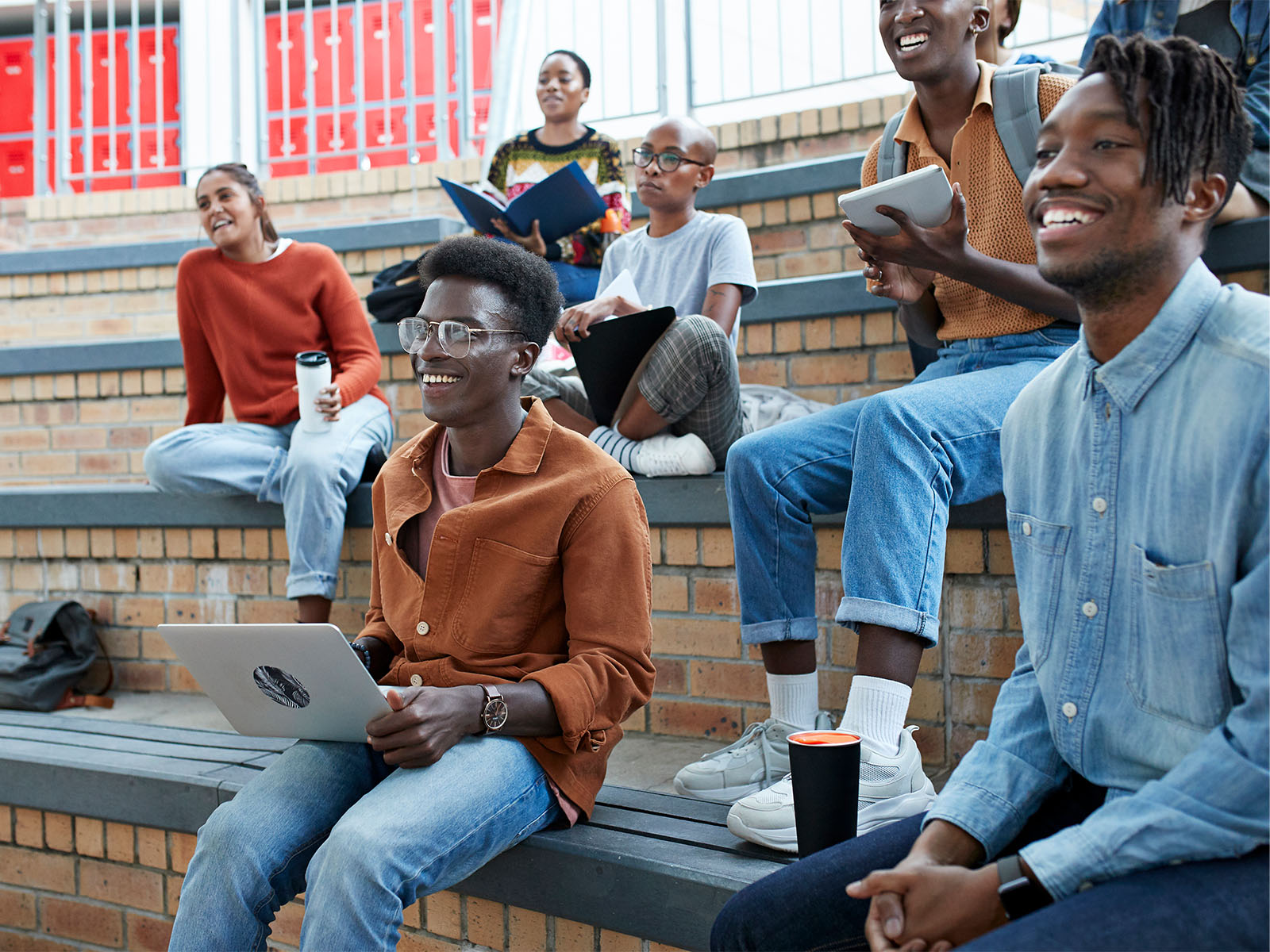
- What is used in RAG?
- How does RAG work?
- Why is Retrieval-Augmented Generation important?
- Integrate RAG into your ML models with HPE
What is used in RAG?
Retrieval-Augmented Generation (RAG) uses a pre-trained retriever to effectively extract important information from big corpora or databases to improve language model creation. This strategy lets the model access more knowledge than pre-training data, resulting in more accurate and informative outputs. RAG dynamically combines external knowledge sources and improves question-answering summarization and content development. RAG might help natural language processing systems provide more contextually rich and accurate outputs by smoothly merging retrieval and production.